[toc]
缓存淘汰
背景
不论是进程内缓存,还是分布式缓存,都无法避免这样一个问题:当我们需要缓存的数据大于物理内存时,那么就需要通过操作系统虚拟内存管理不断地在硬盘和内存中进行页面置换。设想一下:缓存数据越大,那么缺页中断的就越多,导致页面置换就越多,从而磁盘IO增加,从而大大拖垮缓存的性能。所以,虽然操作系统允许我们运行比物理内存更大更多的进程,但是为了兼具缓存性能,我们依然需要适当的控制,来保证我们缓存数据的大小不能超过物理内存,一旦超过就可能会发生页面置换中的磁盘IO
所以,我们可以采取如下一些策略。
- 内存足够时,我们也可以进一步减轻内存负担,例如过期删除、主动清理等
- 内存不足够时,需要通过一些好的策略来做内存数据的淘汰,避免物理内存不足后发生页面置换现象;或者我们直接加内存
在缓存数据的淘汰中,我们需要将数据分为两类:高频热数据和低频冷数据。我们应该尽可能地避免高频热数据被缓存淘汰掉,这样会降低缓存的命中率。所以,我们需要采取一些好的缓存策略来保证我们的缓存命中率。
几种缓存淘汰策略及实现
FIFO先进先出淘汰
缓存满了之后,自动删除较早放入的缓存数据。
1
2
3
4
5
6
7
8
9
10
11
12
13
14
15
16
17
18
19
20
21
22
23
24
25
26
27
28
29
30
31
32
33
34
35
36
37
38
39
40
41
42
43
44
45
46
47
48
49
50
51
52
53
54
55
56
57
58
59
60
61
62
63
64
65
66
67
68
69
70
71
72
73
74
75
|
// @author cold bin
// @date 2023/5/18
package cache
import (
"container/list"
"errors"
"sync"
)
type fifoCache struct {
maxCap uint32 // 缓存允许的最大容量,这里就暂时用个粗略地数字单表允许地容量
list *list.List // 保证fifo
cacheMap map[string]*list.Element // 缓存
lock sync.RWMutex
}
var _ Cache = &fifoCache{}
func NewFifoCache(maxCap uint32) Cache {
return &fifoCache{
maxCap: maxCap,
list: &list.List{},
cacheMap: make(map[string]*list.Element, maxCap),
}
}
func (c *fifoCache) Get(key string) (any, error) {
if v, ok := c.cacheMap[key]; ok {
return v, nil
}
return nil, errors.New("key不存在")
}
// Set 当容量足够时,就可以继续放;当容量不足够时,就按照fifo策略淘汰
func (c *fifoCache) Set(key string, value any) error {
c.lock.Lock()
defer c.lock.Unlock()
// 存在即更新
if v, ok := c.cacheMap[key]; ok {
v.Value = value
c.cacheMap[key] = v
return nil
}
// 是否触发缓存淘汰,这里容量判断(实际上精确地内存占用判断较为困难,因为操作系统不只有物理内存,还有虚拟的内存,只能粗略估计)
if c.list.Len()*10 >= int(c.maxCap) && c.list.Len() > 0 {
// 淘汰最早节点
k := c.list.Back()
delete(c.cacheMap, k.Value.(string))
c.list.Remove(k)
}
// 不存在即添加
c.cacheMap[key] = &list.Element{Value: value}
c.list.PushFront(key)
return nil
}
func (c *fifoCache) Del(key string) error {
c.lock.Lock()
defer c.lock.Unlock()
if e, ok := c.cacheMap[key]; ok {
delete(c.cacheMap, key)
c.list.Remove(e)
return nil
}
return errors.New("key不存在")
}
|
- 优点:实现很简单
- 缺点:先进来的数据可能是热点数据,淘汰掉热点数据,会导致缓存的命中率降低。
LFU最不经常使用
LFU最不经常使用算法,是基于数据的访问频次来对缓存数据进行淘汰的算法。
其淘汰数据依据两点:访问频次
核心:如果数据过去被访问多次,那么将来被访问的频率也就更高,当内存超过最大容量时,淘汰掉访问次数较少的数据。
依然使用队列实现,淘汰部分淘汰掉访问次数最低即可
1
2
3
4
5
6
7
8
9
10
11
12
13
14
15
16
17
18
19
20
21
22
23
24
25
26
27
28
29
30
31
32
33
34
35
36
37
38
39
40
41
42
43
44
45
46
47
48
49
50
51
52
53
54
55
56
57
58
59
60
61
62
63
64
65
66
67
68
69
70
71
72
73
74
75
76
77
78
79
80
81
82
83
84
85
86
87
88
89
90
91
92
93
94
95
96
97
98
99
100
101
102
103
104
105
106
107
108
109
110
111
112
113
114
115
116
117
118
119
120
121
122
123
124
125
126
|
// @author cold bin
// @date 2023/5/18
package cache
import (
"errors"
"sort"
"sync"
)
type lfuCache struct {
maxCap uint32 // 缓存允许的最大容量
counts counts // 记录访问频次的队列
cacheMap map[string]*entry // 缓存
lock sync.RWMutex
}
var _ Cache = &lfuCache{}
type counts []*entry
func (c counts) find(key string) int {
for i, e := range c {
if e.k == key {
return i
}
}
return -1
}
// 更新频次,需要重新调整序列
func (c *counts) update(en *entry, v any, weight int) {
en.v = v
en.weight = weight
// 调整排序
sort.Sort(c)
}
func (c counts) Len() int {
return len(c)
}
func (c counts) Less(i, j int) bool {
return c[i].weight < c[j].weight
}
func (c counts) Swap(i, j int) {
c[i], c[j] = c[j], c[i]
c[i].idx = i
c[j].idx = j
}
type entry struct {
k string
v any
weight int
idx int
}
func NewLfuCache(maxCap uint32) Cache {
return &lfuCache{
maxCap: maxCap,
counts: make([]*entry, 0, 1024),
cacheMap: make(map[string]*entry, maxCap),
}
}
func (c *lfuCache) Get(key string) (any, error) {
c.lock.Lock()
defer c.lock.Unlock()
if v, ok := c.cacheMap[key]; ok {
// 找key并更新访问频次
c.counts.update(v, v.v, v.weight+1)
return v, nil
}
return nil, errors.New("key不存在")
}
func (c *lfuCache) Set(key string, value any) error {
c.lock.Lock()
defer c.lock.Unlock()
// 存在即更新
if e, ok := c.cacheMap[key]; ok {
c.counts.update(e, value, e.weight+1)
return nil
}
// 不存在即新增
e := &entry{
k: key,
v: value,
weight: 0,
idx: -1,
}
c.cacheMap[key] = e
c.counts = append(c.counts, e)
// 容量满了,触发内存淘汰
if c.counts.Len()*10 >= int(c.maxCap) && len(c.counts) > 0 {
idx := len(c.counts) - 1
e := c.counts[idx]
c.counts = c.counts[:idx]
delete(c.cacheMap, e.k)
}
return nil
}
func (c *lfuCache) Del(key string) error {
c.lock.Lock()
defer c.lock.Unlock()
if _, ok := c.cacheMap[key]; ok {
i := c.counts.find(key)
c.counts = append(c.counts[:i], c.counts[i+1:]...)
delete(c.cacheMap, key)
return nil
}
return errors.New("key不存在")
}
|
LRU最近最少使用
最近最少使用缓存淘汰算法,其淘汰最近一段时间最少被访问的缓存数据。我们使用队列可以实现这种淘汰算法,对于访问的元素,移动到链表尾,这样链表头为较旧的元素,当容量满时,淘汰掉链表头元素即可。
1
2
3
4
5
6
7
8
9
10
11
12
13
14
15
16
17
18
19
20
21
22
23
24
25
26
27
28
29
30
31
32
33
34
35
36
37
38
39
40
41
42
43
44
45
46
47
48
49
50
51
52
53
54
55
56
57
58
59
60
61
62
63
64
65
66
67
68
69
70
71
72
73
74
75
76
77
78
79
80
81
82
83
84
85
86
87
88
89
90
91
92
93
94
95
96
97
98
99
100
101
|
// @author cold bin
// @date 2023/5/18
package cache
import (
"container/list"
"errors"
"sync"
)
type lruCache struct {
maxCap uint32 // 缓存允许的最大容量
list []*list.Element // 记录访问顺序
cacheMap map[string]*list.Element // 缓存
lock sync.RWMutex
}
var _ Cache = &lruCache{}
func NewLruCache(maxCap uint32) Cache {
return &lruCache{
maxCap: maxCap,
list: make([]*list.Element, 0, 1024),
cacheMap: make(map[string]*list.Element, maxCap),
}
}
func (c *lruCache) find(key string) int {
for i, k := range c.list {
if k.Value == key {
return i
}
}
return -1
}
// lru核心
func (c *lruCache) swapLast(key string) {
i := c.find(key)
if i == -1 {
panic("i==-1")
}
e := c.list[i]
c.list = append(c.list[:i], c.list[i+1:]...)
c.list = append(c.list, e)
}
func (c *lruCache) Get(key string) (any, error) {
c.lock.Lock()
defer c.lock.Unlock()
if v, ok := c.cacheMap[key]; ok {
// 元素key移到尾部
c.swapLast(key)
return v, nil
}
return nil, errors.New("not found")
}
func (c *lruCache) Set(key string, value any) error {
c.lock.Lock()
defer c.lock.Unlock()
// 存在则更新
if v, ok := c.cacheMap[key]; ok {
v.Value = value
// c.cacheMap[key] = v
c.swapLast(key)
return nil
}
// 容量满时则淘汰头部元素即可
if len(c.list)*10 >= int(c.maxCap) && len(c.list) > 0 {
tmpKey := c.list[0]
c.list = c.list[1:]
delete(c.cacheMap, tmpKey.Value.(string))
}
// 不存在则添加
v := &list.Element{Value: value}
k := &list.Element{Value: key}
c.cacheMap[key] = v
c.list = append(c.list, k)
return nil
}
func (c *lruCache) Del(key string) error {
c.lock.Lock()
defer c.lock.Unlock()
i := c.find(key)
c.list = append(c.list[:i], c.list[i+1:]...)
delete(c.cacheMap, key)
return nil
}
|
上面lru实现的时间复杂度较高。其实可以采用双向链表+哈希表的方式实现O(1)级别的时间复杂度
-
优点:缓存命中率较高
-
缺点:
-
内存占用高
-
缓存污染
当我们在批量读取数据的时候,由于数据被访问了一次,这些大量数据都会被加入到「活跃 LRU 链表」里,然后之前缓存在活跃 LRU 链表(或者 young 区域)里的热点数据全部都被淘汰了,如果这些大量的数据在很长一段时间都不会被访问的话,那么整个 LRU 链表就被污染了。
-
预读失效
操作系统在访问内存数据的时候,如果数据不在内存里,会从磁盘里去加载数据,又因为空间局部性原理,会加载连续的多个块,此时,只有一个块里有热点数据,其余块都没有热点数据,然而还会把lru链表最后的热点数据挤出去。另外几个没有热点数据的块,当然也就预读失效了。
如何解决lru的缓存污染和预读污染问题?
LRU-K
lru-1
数据第一次被访问,加入到访问历史列表;
如果数据在访问历史列表里后没有达到K次访问,则按照LRU淘汰;
当访问历史队列中的数据访问次数达到K次后,将数据索引从历史队列删除,将数据移到缓存队列中,并缓存此数据,缓存队列重新按照时间排序;
缓存数据队列中被再次访问后,重新排序;
需要淘汰数据时,淘汰缓存队列中排在末尾的数据,即淘汰"倒数第K次访问离现在最久"的数据。
1
2
3
4
5
6
7
8
9
10
11
12
13
14
15
16
17
18
19
20
21
22
23
24
25
26
27
28
29
30
31
32
33
34
35
36
37
38
39
40
41
42
43
44
45
46
47
48
49
50
51
52
53
54
55
56
57
58
59
60
61
62
63
64
65
66
67
68
69
70
71
72
73
74
75
76
77
78
79
80
81
82
83
84
85
86
87
88
89
90
91
92
93
94
95
96
97
98
99
100
101
102
103
104
105
106
107
108
109
110
111
112
113
114
115
116
117
118
119
120
121
122
123
124
125
126
127
128
129
130
131
132
133
134
135
136
137
138
139
140
141
142
143
144
145
146
147
148
149
150
151
152
153
154
155
156
157
158
159
160
161
162
163
164
|
// @author cold bin
// @date 2023/5/19
package cache
import (
"container/list"
"errors"
"sync"
)
type lrukCache struct {
maxCap uint32 // 缓存允许的最大容量
k int // 指定多少次访问后移入缓存队列,一般推荐为两次
history []*entry1 // 访问历史记录,只有访问次数大于等于k的数据才会被缓存,其余数据按照lru淘汰。采用链表更好点
list []*list.Element // 缓存里的key顺序,最近访问的元素放到队列的头部,越往后就是越久的元素,按照lru方式淘汰
cacheMap map[string]*list.Element // 缓存
lock sync.RWMutex
}
var _ Cache = &lrukCache{}
type entry1 struct {
k string
v any
count int // 访问次数
}
// 判断key在哪个队列里:-1->history;1->list
func (c *lrukCache) judge(key string) (idx int, ans int) {
for i, e := range c.history {
if e.k == key {
return i, -1
}
}
for i, e := range c.list {
if e.Value.(string) == key {
return i, 1
}
}
return -1, 0
}
// list里最近访问的元素放到list后面
// 切片这里时间复杂度较高,采用链表的话,更好点
func (c *lrukCache) placeLruk(idx int) {
e := c.list[idx]
c.list = append(c.list[:idx], c.list[idx+1:]...)
c.list = append(c.list, e)
}
// history里最近访问的元素放到history后面
func (c *lrukCache) placeHistory(idx int) {
e := c.history[idx]
c.history = append(c.history[:idx], c.history[idx+1:]...)
c.history = append(c.history, e)
}
// 访问次数达到k的元素从history移到list,并将存入缓存
func (c *lrukCache) historyMoveToList(hidx int) {
e := c.history[hidx]
// 删除
c.history = append(c.history[:hidx], c.history[hidx+1:]...)
// 放到list末尾
c.list = append(c.list, &list.Element{Value: e.k})
// 放入缓存
c.cacheMap[e.k] = &list.Element{Value: e.v}
}
func (c *lrukCache) Get(key string) (any, error) {
c.lock.Lock()
defer c.lock.Unlock()
idx, ans := c.judge(key)
switch ans {
case 1: // list
// 将新访问的缓存移到lru后面
c.placeLruk(idx)
return c.cacheMap[key].Value, nil
case -1: // history
// 访问history,如果访问次数达到k,移到list;否则按照lru规则
c.history[idx].count++
// 先记录值
v := c.history[idx].v
if c.history[idx].count == c.k { // 可以放list和缓存了
c.historyMoveToList(idx)
} else { // lru规则
c.placeHistory(idx)
}
return v, nil
case 0:
return nil, errors.New("not found")
default:
return nil, errors.New("not known error")
}
}
func (c *lrukCache) Set(key string, value any) error {
c.lock.Lock()
defer c.lock.Unlock()
idx, ans := c.judge(key)
switch ans {
case 1: // list
// 更新缓存和list
c.cacheMap[key].Value = value
c.placeLruk(idx)
return nil
case -1: // history
// 更新history
c.history[idx].count++
if c.history[idx].count == c.k { // 可以放list和缓存了
c.historyMoveToList(idx)
} else { // lru规则
c.placeHistory(idx)
}
return nil
case 0: // 不存在即添加到history后,并按照lru淘汰
// 是否触发缓存淘汰
if (len(c.history)+len(c.list))*10 >= int(c.maxCap) {
c.history = c.history[1:]
// 没有缓存
}
// 添加
e := &entry1{k: key, v: value}
c.history = append(c.history, e)
return nil
default:
return errors.New("not known error")
}
}
func (c *lrukCache) Del(key string) error {
c.lock.Lock()
defer c.lock.Unlock()
idx, ans := c.judge(key)
switch ans {
case 1: // list
// 删除list
c.list = append(c.list[:idx], c.list[idx+1:]...)
// 删除缓存
delete(c.cacheMap, key)
return nil
case -1: // history
c.history = append(c.history[:idx], c.history[idx+1:]...)
return nil
default:
return errors.New("not found")
}
}
|
LRU其实就是LRU-1,意思就是最近使用过1次的数据,就加入缓存。这样做可能会导致这样的情况发生:近期前有大量访问,但是近期没有访问的数据,那么这些数据就可能会被LRU规则淘汰掉,但这些数据还是有可能在近期后被访问。这样就造成某些偶尔发生的缓存命中率低的现象。
LRU-K策略指的是,只有数据被访问k次后,才能被加入缓存里,这样可以降低缓存污染(减少冷数据加入缓存的情况),从而提高缓存命中率。
其实linux操作系统中page cache的缓存淘汰策略里也是通过将将lru算法改进为lru-2算法提高进入active list的lru缓存的门槛,从而降低了缓存污染严重性。
2Q
2Q(Two Queue)缓存淘汰算法是一种基于 LRU(Least Recently Used)和 FIFO(First In First Out)算法的混合算法。其主要思想是将缓存分为两个队列:Q1 和 Q2。
当数据第一次访问时,2Q算法将数据缓存在FIFO队列里面,当数据第二次被访问时,则将数据从FIFO队列移到LRU队列里面,两个队列各自按照自己的方法淘汰数据。
1
2
3
4
5
6
7
8
9
10
11
12
13
14
15
16
17
18
19
20
21
22
23
24
25
26
27
28
29
30
31
32
33
34
35
36
37
38
39
40
41
42
43
44
45
46
47
48
49
50
51
52
53
54
55
56
57
58
59
60
61
62
63
64
65
66
67
68
69
70
71
72
73
74
75
76
77
78
79
80
81
82
83
84
85
86
87
88
89
90
91
92
93
94
95
96
97
98
99
100
101
102
103
104
105
106
107
108
109
110
111
112
113
114
115
116
117
118
119
120
121
122
123
124
125
126
127
128
129
130
131
132
133
134
135
136
137
138
139
140
|
// @author cold bin
// @date 2023/5/19
package cache
import (
"container/list"
"errors"
"sync"
)
type twoQueue struct {
maxCap uint32 // 缓存允许的最大容量
fifoList []*list.Element // fifo
lruList []*list.Element // lru
cacheMap map[string]*list.Element // 缓存
lock sync.RWMutex
}
var _ Cache = &twoQueue{}
func NewTwoQueue(maxCap uint32) Cache {
return &twoQueue{
maxCap: maxCap,
fifoList: make([]*list.Element, 0, 1024),
lruList: make([]*list.Element, 0, 1024),
cacheMap: make(map[string]*list.Element, 1024),
}
}
func (c *twoQueue) placeLru(idx int) {
e := c.lruList[idx]
c.lruList = append(c.lruList[:idx], c.lruList[idx+1:]...)
c.lruList = append(c.lruList, e)
}
// 判断key存在与哪个队列。-1->fifo;1->lru
func (c *twoQueue) judge(key string) (idx int, ans int) {
for i, k := range c.fifoList {
if k.Value.(string) == key {
return i, -1
}
}
for i, k := range c.lruList {
if k.Value.(string) == key {
return i, 1
}
}
return -1, 0
}
func (c *twoQueue) Get(key string) (any, error) {
c.lock.Lock()
defer c.lock.Unlock()
if _, ok := c.cacheMap[key]; ok {
// 如果访问数据在fifo队列里,就移到lru队列;如果在lfu队列里,就移到lru尾部
idx, ans := c.judge(key)
switch ans {
case 1: // lru
c.placeLru(idx)
case -1: // fifo
// 删除
k := c.fifoList[idx]
c.fifoList[idx] = nil
c.fifoList = append(c.fifoList[:idx], c.fifoList[idx+1:]...)
// 移动到lru尾部
c.lruList = append(c.lruList, k)
// 是否触发lru内存淘汰
if (len(c.lruList)+len(c.fifoList))*10 >= int(c.maxCap) && len(c.lruList) > 0 {
tmpKey := c.lruList[0]
c.lruList = c.lruList[1:]
delete(c.cacheMap, tmpKey.Value.(string))
}
case 0:
return nil, errors.New("not exist")
default:
return nil, errors.New("not known error")
}
}
return nil, errors.New("not exist")
}
func (c *twoQueue) Set(key string, value any) error {
c.lock.Lock()
defer c.lock.Unlock()
if _, ok := c.cacheMap[key]; ok {
// 存在就更新:如果访问数据在fifo队列里,就移到lru队列;如果在lru队列里,就移到lru尾部
idx, ans := c.judge(key)
switch ans {
case 1: // lru
c.placeLru(idx)
case -1: // fifo
// 删除
k := c.fifoList[idx]
c.fifoList[idx] = nil
c.fifoList = append(c.fifoList[:idx], c.fifoList[idx+1:]...)
// 是否触发lru内存淘汰
if (len(c.lruList)+len(c.fifoList))*10 >= int(c.maxCap) && len(c.lruList) > 0 {
tmpKey := c.lruList[0]
c.lruList = c.lruList[1:]
delete(c.cacheMap, tmpKey.Value.(string))
}
// 移动到lru尾部
c.lruList = append(c.lruList, k)
case 0:
return errors.New("not exist")
default:
return errors.New("not known error")
}
}
// fifo是否触发缓存淘汰
if (len(c.fifoList)+len(c.lruList))*10 >= int(c.maxCap) && len(c.fifoList) > 0 {
// 淘汰最早节点
k := c.fifoList[0]
delete(c.cacheMap, k.Value.(string))
c.fifoList = c.fifoList[1:]
}
// 新增则加入fifo队列
c.cacheMap[key] = &list.Element{Value: value}
c.fifoList = append([]*list.Element{&list.Element{Value: key}}, c.fifoList...)
return nil
}
func (c *twoQueue) Del(key string) error {
//TODO implement me
panic("implement me")
}
|
- 优点:更快,一般来说可以实现O(1)级别的淘汰
- 缺点:belady现象:物理内存增加反而导致缓存命中率下降
应用与举例
redis中的近似LRU策略
当实际内存超出 maxmemory
时,就会执行一次内存淘汰策略,具体何种内存淘汰策略,可在maxmemory-policy
中进行配置。Redis 提供了如下几种策略:
noeviction
:不会继续服务写请求 (DEL 请求可以继续服务),读请求可以继续进行。这样可以保证不会丢失数据,但是会让线上的业务不能持续进行。这是默认的淘汰策略。
volatile-lru
:尝试淘汰设置了过期时间的 key,最少使用的 key 优先被淘汰。没有设置过期时间的 key 不会被淘汰,这样可以保证需要持久化的数据不会突然丢失。
volatile-ttl
:跟上面一样,除了淘汰的策略不是 LRU,而是 key 的剩余寿命 ttl 的值,ttl 越小越优先被淘汰。
volatile-random
:跟上面一样,不过淘汰的 key 是过期 key 集合中随机的 key。
allkeys-lru
:区别于 volatile-lru
,这个策略要淘汰的 key 对象是全体的 key 集合,而不只是过期的 key 集合。这意味着没有设置过期时间的 key 也会被淘汰。
allkeys-random
:跟上面一样,不过淘汰的策略是随机的 key。
volatile-xxx
策略只会针对带过期时间的 key 进行淘汰,allkeys-xxx
策略会对所有的 key 进行淘汰。
如果你只是拿 Redis 做缓存,那应该使用 allkeys-xxx
,客户端写缓存时不必携带过期时间。
如果你还想同时使用 Redis 的持久化功能,那就使用 volatile-xxx
策略,这样可以保留没有设置过期时间的 key,它们是永久的 key 不会被 LRU 算法淘汰。
redis中的lru:
在 volatile-lru
策略中,Redis 并没有采用经典的 LRU 算法,而是采用了一种近似 LRU 算法。至于为什么会不是。主要有以下几点:
- 内存占用高(除了缓存数据,需要额外引入链表来记录key的访问顺序,以实现lru策略)
- 大量节点的访问,会带来链表或队列中节点频繁的移动,较为损耗性能
- 传统lru会出现缓存污染问题和预读失效问题(当然,redis并没有预读缓存)
那么针对以上两个问题,近似lru策略这样解决:
-
近似 LRU 算法中,是在现有数据结构的基础上,使用随机采样法来淘汰元素,避免额外的内存开销。(就是每次淘汰的数据并不是全部的key,而是采取的样本)
-
近似 LRU 算法中,Redis 给每个 key 增加了一个额外的小字段(长度为 24 个 bit),存储了元素最后一次次被访问的时间戳。Redis 会对少量的 key 进行采样,并淘汰采样的数据中最久没被访问过的 key。这也就意味着 Redis 无法淘汰数据库最久访问的数据。
-
Redis LRU 算法有一个重要的点在于,它可以更改样本数量(修改 maxmemory-samples
属性值)来调整算法的精度,使其近似接近真实的 LRU 算法,同时又避免了内存的消耗,因为每次只需要采样少量样本,而不是全部数据。
bigcache中的fifo策略
完完全全的fifo策略,淘汰的时候会淘汰掉最早的数据,但是容易出现缓存命中率不高的问题。总而言之,fifo只考虑了时间维度因素,并没有考虑到访问频率因素。
当然选取fifo策略,相较于lru、lfu等策略实现起来更简单,而且也更快,内存占用也更低
memcached中的lru策略
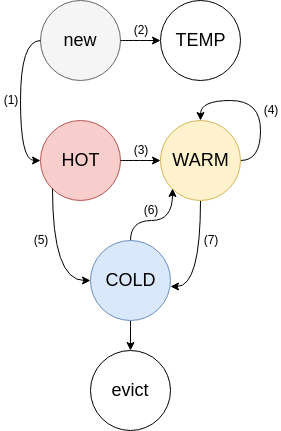
memcached的缓存淘汰策略是受2Q设计影响的修改LRU(Segmented LRU,分段LRU)。主要是借鉴了2q策略中的数据分类,memcached将数据分为以下几类:
- HOT queue:如果一个 item 的过期时间(TTL)很短,会进入该队列,在 HOT queue 中不会发生 bump,如果一个 item 到达了 queue 的 tail,那么会进入到 WARM 队列(如果 item 是 ACTIVE 状态)或者 COLD 队列(如果 item 处于不活跃状态)。
- WARM queue:如果一个 item 不是 FETCHED,永远不会进入这个队列,该队列里面的 item TTL 时间相对较长,这个队列的 lock 竞争会很少。该队列 tail 处的一个 item 如果再一次被访问,会 bump 回到 head,否则移动到 COLD 队列。
- COLD queue:包含了最不活跃的 item,一旦该队列内存满了,该队列 tail 处的 item 会被 清除。如果一个 item 被激活了,那么会异步移动到 WARM 队列。
- TEMP queue:该队列中的 item TTL 通常只有几秒,该列队中的 item 永远不会发生 bump,也不会进入其他队列,节省了 CPU 时间,也避免了 lock 竞争。
“bump”是memcached中的一个标记,用于标记某个键值对不应该被淘汰。
总结
- 根据业务需求选择合适的算法。不同的业务场景对缓存的需求不同,因此需要根据实际情况选择合适的缓存淘汰算法。
- 避免频繁清除缓存。频繁清除缓存会导致缓存命中率降低,从而影响系统的性能。因此,在选择缓存淘汰算法时,需要考虑清除缓存的频率。
- 配置合适的缓存容量。缓存容量过小会导致缓存命中率降低,而缓存容量过大会浪费系统资源。因此,需要根据实际情况配置合适的缓存容量。
- 考虑多级缓存。多级缓存可以提高缓存命中率,从而提高系统的性能。例如,可以比如bigcache+redis的方式构建多级缓存。
- 使用缓存预热技术。缓存预热技术可以在系统启动时将常用的数据预先加载到缓存中,从而提高缓存命中率。